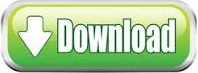

- #Valentina studio only 1000 entries update#
- #Valentina studio only 1000 entries full#
- #Valentina studio only 1000 entries code#
As part of applying this parameter, we also set the parameters wal_level, max_wal_senders, max_replication_slots, and max_connections. Either duplicate or modify the parameter group directly with the following.ġ.- Set the rds.logical_replication static parameter to 1. Since I don’t consider myself smarter than people that write AWS documentation, I’ll copy-paste some of their instructions below 🤷♂.įind your RDS instance, look up the parameter group that this instance applies. Feel free to read one or the other, or even better, both 😄. The second one, building the S3 data-lake for other services to use. The first one will be the steps to replicate changes directly to Redshift. For more info, click this link.įor this post to be more “democratic”, I’ll divide it into 2 sections. This enables you to subscribe anything to that topic, for example, multiple lambdas. (Optional) An SNS topic subscribed to the same event of object creation.A Lambda that triggers every time an object is created in the S3 bucket mentioned above.An S3 bucket used by DMS as a target endpoint.A DMS (Database Migration Service) instance replicating on-going changes to Redshift and S3.Our current architecture consists of the following: What you came here forĪrchitecture diagram - Made with Lucidchart Long story short, the function could still be terminated unsuccessfully due to not enough time to process the huge transaction. We solved this by setting true to the property write_in_chunks of the logical decoding plugin, we used ( wal 2json ), enabling us to partition the incoming JSON log.
#Valentina studio only 1000 entries full#
We found out that, in these cases, the way the records came from the logical decoding were huge rows full of chunks of changes of the tables involved, causing the function to die of lack of memory every time it tried to load it. It seemed to be all safe and sound until a production process updated more rows than usually expected. We tried to set a Lambda to consume every 15 minutes the Wal log of a replication slot of our Postgres database, and send it to a Kinesis Firehose data stream. Furthermore, there isn’t much detailed documentation or clear examples for this service IMO.
#Valentina studio only 1000 entries code#
Since you can’t use code here, it became unmaintainable quickly.
#Valentina studio only 1000 entries update#
Although the process of building an ETL was rather easy, there were a bunch of workarounds that we had to take in order for it to be effective - remember that we have to update every change whether it be an insertion, a deletion or an update. We started by using AWS Data Pipeline, a UI based service to build ETLs between a bunch of data sources. Many things to take into account, right? Testing solutionsīeing a small team of 2 people, the mighty “Data Team”, we get it easy to try and test new things, especially architectures. In other words, we had to build a data-lake accessible for consumption by any service to perform syncing operations on-demand. The problem is, both products must be synced in order for Conciliación to use transactions extracted by Card. This one uses the NoSQL Apache Cassandra database to store and process its huge data. The company’s developing its second major product, IncreaseConciliación. Argentina’s Congress - Photo by Sander Crombach on Unsplash
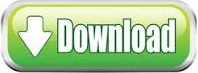